Adaptive-Grained CO2 Emission Estimation Using Spatiotemporal Cells with Heterogeneous Vehicles
project
Jan 2021
This study presents an adaptive-grained method for estimating CO2 emissions using spatiotemporal cells, focusing on heterogeneous vehicles. It leverages PEMS technology to collect emissions data and applies DNN and LSTM models for carbon emission prediction in varying grid sizes, aimed at improving accuracy and adaptability in different urban scenarios.
- CO2 Emissions
- Heterogeneous Vehicles
- PEMS Technology
- DNN
- LSTM
- + more
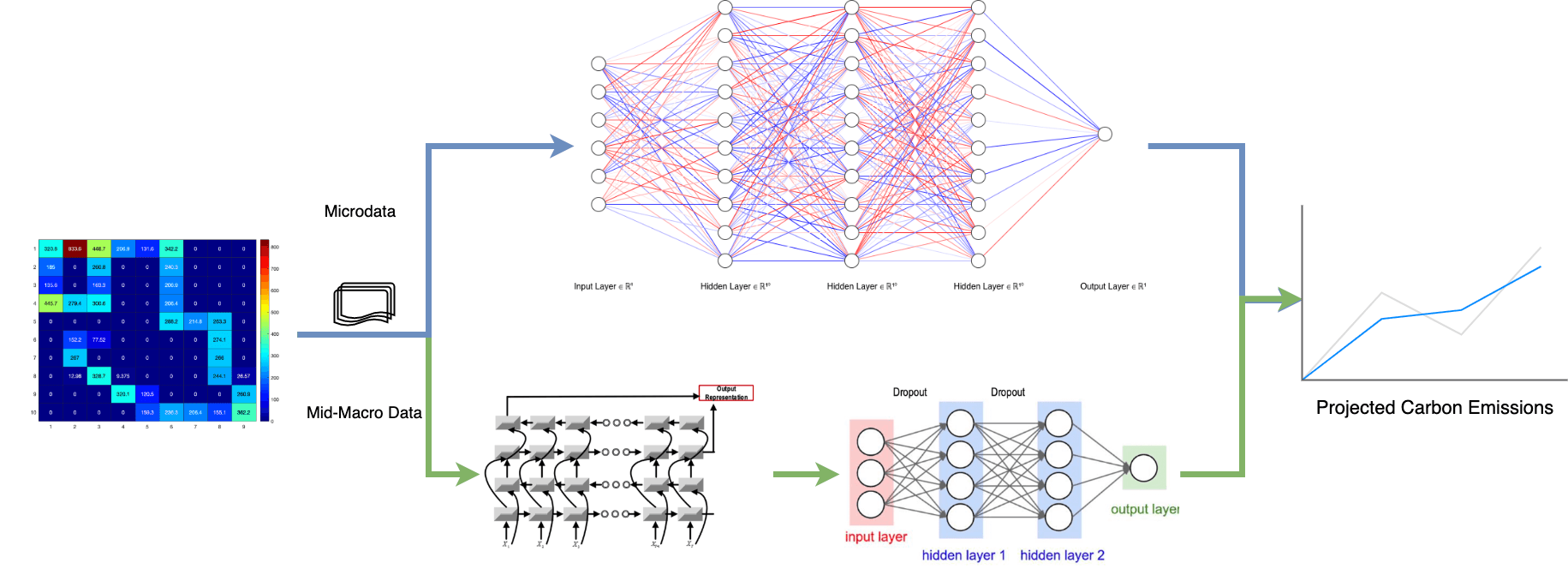